Improve the data supervision system, on the one hand, it is necessary to address the issues of insufficient public data exploration, low quality, and lack of openness; on the other hand, it is necessary to address the issues of unclear ownership, inadequate protection, and poor circulation of enterprise and personal data.
From September 5th to 7th, the 6th Bund Financial Summit "Responding to a Changing World," co-hosted by the China Finance 40 Forum (CF40) and the China Center for International Economic Exchanges (CCIEE), was held in Huangpu District, Shanghai.
Global financial and political leaders, institutional executives, and academic leaders gathered at the Bund to seek strategies for the development of a changing world and explore new paths for global cooperation and development.
At the sub-forum on September 6th, Li Lihui, former president of the Bank of China, introduced that China's non-public data currently has issues with poor circulation.
For example, the number of our mobile payment customers is now almost over 900 million, and mobile payment platforms are the main entrances for data.
Advertisement
However, data sharing between financial institutions and mobile platforms has not yet formed a more mature model.
"We still have the problem of administrative departmental segmentation of public data, which still affects data sharing and the realization of data value.
For example, some data involving residents and enterprises are distributed in different departments such as financial institutions, financial supervision, taxation, customs, and business management, and there is administrative data segmentation, which affects the development of data value."
Li Lihui said.
At the same time, "to comprehensively improve the productivity of data elements and maximize the value of data resources, it is required to efficiently allocate data element resources.
Due to the different ownership of data, the allocation of data element resources should also adopt a diversified composite approach.
A single market-oriented path or a single administrative path is difficult to achieve the optimal allocation efficiency."
Li Lihui believes.
How to solve data segmentation, data flow, and improve data quality?
Around issues related to data and intelligent finance, Li Lihui accepted an exclusive interview with "Finance and Economics".
"Finance and Economics": You mentioned that the value of data lies in its true becoming a factor of production.
It is necessary to break the administrative departmental ownership of public data.
Establish a unified standard and interconnected public data application system, establish a credit system for data sharing, and form infrastructure that can support the development of the digital economy.
Where do you think the difficulty lies in data becoming a factor of production?
How to break data barriers and achieve data sharing?
Li Lihui: The main difficulty faced by data becoming a factor of production is the "both and" dilemma: it is necessary to protect data privacy and ensure data security, and at the same time, promote data flow and explore data value.
China relies on the advantages of population size, market size, and the first development of mobile services, and has formed a global leading supply pattern of data resources in the fields of market transactions and citizen behavior.
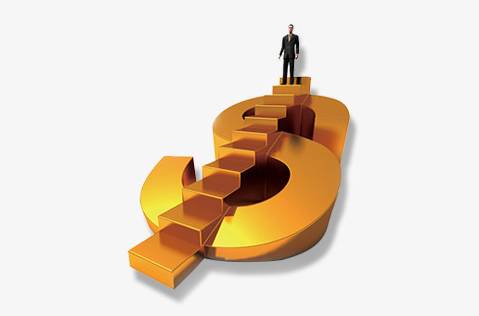
The huge amount of market transaction and citizen behavior data is a precious resource for the development of China's digital economy and digital finance.
China has been promoting data sharing for many years.
Some provinces and cities have established big data centers and have done a lot of work in data concentration, data organization, data mining, and data sharing.
For example, the big data group in Fujian Province has comprehensively promoted the convergence and sharing of data in the province, established a personal database of 70 million and an enterprise database of 8 million, established a multi-dimensional scoring system for credit data of small and micro enterprises, established a special area for transportation and meteorological data, realized data empowerment in thousands of industries, and supported and promoted the digital transformation and development of the financial industry.
Despite this, our data sharing is still lagging, and the data gap still exists.
For example, financial and behavioral data involving residents and enterprises are scattered in different local systems such as financial institutions, financial supervision, business management, taxation, and customs, with a low level of sharing, affecting the in-depth development of data value.
For example, the scale of China's mobile payment users has reached 900 million, and digital payment has become the main data entrance.
Internet platforms have a large-scale personal and enterprise data, but the data association and data sharing between internet platforms and financial institutions have not yet reached a mature model, and the value of data has not been fully explored.
The main reason is that the institutional boundaries for protecting data privacy and ensuring data security are not clear and not clear.
The relevant administrative departments would rather strictly manage and dare not loosen the binding, and the data resources are many and the applications are few.
Therefore, to break data barriers, it is necessary to start with institutional innovation.
First, clarify the principles, emphasize the coordination and unity of achieving multiple goals such as protecting personal privacy, protecting information security, exploring data value, and improving economic efficiency, and do not generalize; second, implement responsibilities, and require national administrative departments to correctly distinguish the boundaries of privacy protection within their respective fields, correctly grasp the intensity of data security control, formulate industry norms for data sharing, and do not shirk responsibilities; third, improve in practice, first let the data elements move, live, and use, correct errors in the development process, gradually improve industry norms, and when mature, elevate industry norms to regulations and laws, and do not stop.
In the construction of the social credit system, it is necessary to establish a credit system for cross-regional data sharing, integrate data resources from different departments, implement unified standards, collect financial business, industrial and commercial registration, tax payment, international trade, market integrity, and other information data of enterprises and individuals, focus on accumulating credit records for small and micro enterprises, give credit marks, achieve credit value-added, and promote the development of inclusive financial business.
"Finance and Economics": How to resolve the issue of unclear data ownership from the regulatory level?
Li Lihui: The Third Plenary Session of the Central Committee proposed: to build and operate the national data infrastructure, promote data sharing; to accelerate the establishment of data property rights identification, market trading, rights and interests distribution, and interest protection systems; the 20 data requirements: to improve the structural separation system of data property rights, and to establish a classified and graded rights and authorization system for public data, enterprise data, and personal data.
On the regulatory level, on the one hand, it is necessary to solve the problems of insufficient public data exploration, low quality, and lack of openness, and on the other hand, it is necessary to solve the problems of unclear ownership, inadequate protection, and poor circulation of enterprise and personal data.
First, the exploration, desensitization, and open sharing of public data.
Western developed countries such as the United States have long-term development and accumulation of data resource advantages in the fields of knowledge and academia.
China's high-quality cultural, scientific and technological, and visual data resources are relatively scarce, and high-quality data sets that meet the needs of AI large models are far from enough.
It is necessary to accelerate data desensitization and expand the open application of public data.
Personal information collected and stored by institutions with public attributes such as government administrative departments, public service institutions, social organizations, and public welfare organizations should be desensitized to eliminate personal and enterprise marks.
The state establishes a centralized and unified public database and an interconnected public data application system to break the data islands.
Public data should be provided to the society in the form of models, verification, and other products and services according to the requirements of "original data does not leave the domain, data is available but not visible", and increase the supply and use range.
Public data should be fully exploited, used for macroeconomic management and planning, used for structural reforms on the supply side and demand side, used for the layout and process improvement of the industrial chain, used for urban intelligent management systems, and used for inclusive finance; at the same time, public data should be used for the optimization of products and services of manufacturers, and for the optimization of procurement and logistics of dealers.
It is necessary to accelerate the data exploration of public documents and establish a specialized public database.
Libraries, colleges and universities, scientific research institutions, and administrative departments have a large number of public documents with cultural value, technical value, and economic value, which should be planned overall, classified, and cleaned in batches for dataization, to explore high-quality data sets and enrich the public database.
Second, the rights confirmation, protection, and joint use of enterprise data and personal data.
For the data collected and processed by various market entities in production and business activities that do not involve personal information and public interests, the market entity's ownership, use rights, and income rights to the data should be confirmed.
What needs to be carefully grasped is the data that carries personal information collected and processed by market entities in production and business activities.
Data processors must collect, hold, host, and use data within the scope of personal authorization and legal permission, improve the personal information protection mechanism, and standardize the collection and use of personal information.
At the same time, innovative technical means are needed to promote the anonymization processing of personal information, to ensure information security and personal privacy when using personal information data.
The financial industry is a data-intensive industry, and the development of inclusive financial business is the policy direction of the state.
The amount and quality of data possessed by different financial institutions and their ability to mine data can largely determine the actual ability of a financial institution to operate inclusive financial business, including the quantity and quality of inclusive financial business, risk costs, and operating costs.
It is necessary to accelerate the new model of "joint use and shared benefits" of non-public data in a market-oriented manner, support financial institutions to establish a market-oriented data sharing mechanism with "data big households" such as internet platform companies, transportation companies, logistics companies, data analysis and processing companies, credit agencies, administrative departments, and public service institutions, to provide data support for inclusive financial business, and promote the financial industry to provide efficient financial services for small and micro enterprises.
"Finance and Economics": The "Opinions of the Central Committee of the Communist Party of China and the State Council on Building a Data Basic System to Better Play the Role of Data Elements" proposes to establish a data property right system that guarantees rights and interests and uses in compliance with regulations.
What do you think are the elements of a reasonable data property right system?
How to promote the formation of this system?
The 20 data points define the basic system of data as the data property right system, the circulation and trading system of data elements, the income distribution system of data elements, and the governance system of data elements.
The data property right is divided into the ownership of data resources, the right to use and process data, and the right to operate data products.
A perfect data property right system should be based on the optimization of the allocation of data element resources and the maximization of the value of data elements.
Since data elements have different properties and ownership, the best path to maximize value is also different.
The maximization of the value of written data depends on the right to the market, the maximization of the value of personal data depends on privacy protection and integrated application, and the maximization of the value of public data depends on transparency and resource sharing.Establishing a digital asset market is conducive to improving the system of data property rights.
The era of the digital economy requires a market for digital assets.
The digital asset market includes digital financial assets, physical assets, and assetized patent data, and copyright data.
Digital financial assets can be authenticated, priced, and traded in the digital asset market, achieving the transfer of ownership.
Digital physical assets can be authenticated, priced, and traded for ownership or usage rights in the digital asset market.
Assetized patent data and copyright data refer to the digital asset forms of "patents" and "works" defined by laws such as the "Patent Law" and the "Copyright Law," which are part of intellectual property rights.
Inventions and innovations that have novelty, creativity, and practicality can be granted patent rights, and patent application rights and patent rights can be transferred.
Works include original intellectual achievements in the fields of literature, art, and science that can be expressed in a certain form, including literary works, oral works, artistic works, fine arts, architectural works, photographic works, audiovisual works, engineering design drawings, product design drawings, and other graphic works and model works, computer software, and other intellectual achievements that meet the characteristics of works.
The ownership or usage rights of copyright data can be authenticated, priced, and traded in the digital asset market.
In the digital asset market, authentication is a key link.
Applying digital technologies such as blockchain and artificial intelligence to establish a digital trust mechanism has advantages over traditional asset markets.
First, it forms a trustworthy bond in environments where trust is unknown or weak, saving time and cost required for credit formation and enhancing commercial credit.
Second, it establishes a credible authentication tool in a wide-area, high-speed network, solving the problem of rights confirmation for patents and patent holders, copyright data and copyright owners, achieving credible data, credible property rights, credible authorization, credible contracts, and credible legal persons.
This will be beneficial for fully exploring the commercial value of data resources.
Innovation and application of digital technology are building a distributed business model characterized by peer-to-peer, mutual trust, and win-win.
Parties with data resource advantages and other parties with advantages in capital, technology, labor, land, customers, channels, and other elements can carry out multi-party cooperation and benefit exchange based on resource sharing, gather comparative advantages, reduce opportunity costs, and benefit from exchange and cooperation.
Distributed business can break away from the local optimization of maximizing individual interests under the traditional business model, create Pareto optimization through resource coordination, pursue structural coordination in various links of the industry chain and supply chain, and achieve global optimization.
"Finance and Economics": You mentioned AI trust and AI security issues before.
After the widespread application of AI technology, problems such as AI-generated false content and AI manipulation of human behavior have also emerged.
What is a reasonable AI trust system and AI regulatory system?
What aspects should be legislated and regulated?
Li Lihui: AI technology innovation and application have brought new challenges, and it is necessary to focus on controlling AI alignment, AI falsehood, and AI manipulation.
"AI alignment" refers to the goal of AI systems must be consistent with human values and fundamental interests, and this concept has been recognized by global principles.
The problem is that human values and fundamental interests may not be consistent, and there are differences and even conflicts in the values and fundamental interests of different entities and groups.
In the context of geopolitics, AI alignment may become a technical tool for the export of hegemonic country values.
"AI falsehood" refers to the use of generative algorithms such as deep learning and virtual reality to create highly realistic images, audio, and video, which can blur the boundary between the real and the false, and even resist general technical identification, and has been used to carry out economic fraud and to defame personal reputation and corporate reputation.
"AI manipulation" refers to the use of highly realistic images, audio, and video to manipulate negative public opinion, smear political opponents, destroy political trust, destabilize society, and intensify social contradictions.
On July 18, 2023, at the United Nations Security Council, United Nations Secretary-General Guterres said that artificial intelligence is playing a role in many fields, but it may be used by malicious people, including using it for terrorism, inciting hatred and violence.
Therefore, we need to implement digital economy governance that emphasizes both security and efficiency.
First, strictly control AI falsehood and AI manipulation.
The state establishes an AI trust system and an AI regulatory system, clearly prohibits AI forgery and AI fraud at the legislative and law enforcement levels, and grants qualified enterprises AI trust marks; improve deep synthesis content identification technology, provide public services against AI falsehood, and establish a firewall at the national level to prevent AI manipulation.
Second, actively participate in the formulation of global AI standards, integrate Chinese factors into the global system construction of "AI alignment," and offset the negative impact of Western hegemony.
Third, pay attention to the potential risks of AI software open source.
Credible open source is a feasible path for digital technology innovation, and we cannot refuse open source.
OpenAI's shift from open source to closed source of GPT should be to maintain a technological lead, and Meta's open source of Llama3 is to compete for the market.
It must be noted that the inherent system binding and technical dependence of open source algorithms, first, the technical ceiling of open source models is difficult to surpass, second, models pre-trained with foreign data may not meet local needs, and third, the cessation of open source may cause a technological gap.
Software open source collaboration rules include public disclosure of source code, allowing derivative works, and maintaining the integrity of the author's source code.
In the open source pattern, it is necessary to establish software security technical standards and software review certification systems to intercept open source software with safety hazards; to increase investment, increase policy support, prioritize the development and use of software and models with independent intellectual property rights, and promote the progress and growth of China's software technology industry.
"Finance and Economics": How to use technology to improve financial supervision?
How to systematically and effectively promote digital financial innovation, and balance innovation and risk in the financial field?
Li Lihui: For intelligent financial innovation, a prudent view believes that investing immature artificial intelligence technology into the high-risk financial field may amplify existing risks and generate new risks.
I believe that the technical defects that the financial industry must focus on solving include model discrimination, algorithm convergence, weak privacy protection, and dependence on key third parties.
Model discrimination refers to the collective bias, bad reviews, and policy restrictions against certain groups in the output results of the model; algorithm convergence refers to the same market trend predictions and the same market trading strategies executed due to the convergence of algorithm logic in intelligent financial programs such as quantitative trading in different institutions, leading to unilateral market transactions, which may trigger market crises of sharp rises and falls.
Intelligent financial innovation must be based on financial security, achieve financial equality in line with ethical standards, ensure financial efficiency in line with safety standards, and create an innovative model in line with economic laws.
First, the integration and development of industry-level vertical models and enterprise-level vertical models.
Pre-training industry-level vertical models with massive data and continuously optimizing them, and then adjusting differentiated applications according to different needs, customizing enterprise-level vertical models, can effectively reduce the marginal cost of developing vertical models and expand the application range of vertical models.
The financial industry covers different fields such as commercial banks, insurance, securities investment, and wealth management.
The needs for financial products, financial services, and financial management in the same field are similar, and adopting the technical route of integrating and developing industry-level vertical models and enterprise-level vertical models, while choosing the appropriate business model, is conducive to achieving high-efficiency, low-input, and personalized financial innovation needs.
Second, build a safe and efficient intelligent financial innovation system.
Overly strict regulation may suppress innovation and industrial development.
The principles that can be considered are "high, middle, initial, and small," "high" is to occupy the technological highland, "middle" is the world-leading Chinese solution, "initial" is the ability to eliminate risks at the embryonic stage, and "small" is to minimize the probability and cost of risks.
This requires accelerating the innovation of intelligent financial supervision, saving supervision costs and the costs of being supervised.
For example, formulating laws and regulations, clarifying the responsibility boundaries of all participants in intelligent finance, including the basic principles of intelligent financial supervision, the responsibilities and powers of regulatory agencies, and the norms of intelligent financial business of financial institutions; establishing a penetrating, integrated, and cross-regional intelligent financial collaborative supervision system to achieve information sharing; establishing an intelligent financial technology review and certification system, improving the testing platform, tools, standards, and methods of AI large models; establishing an intelligent financial risk analysis and monitoring system, identifying, assessing, and giving early warnings of abnormal transactions and market manipulation in a timely manner, actively preventing systemic risks; actively participating in the construction of international rules of the digital economy and general standards of digital technology, strengthening international regulatory cooperation and exchanges in intelligent finance, striving for China's voice in the construction of international rules of the digital economy, and striving for China's "positioning rights" in the construction of general standards of digital technology.